KGML-ag: a modeling framework of knowledge-guided machine learning to simulate agroecosystems: a case study of estimating N2O emission using data from mesocosm experiments
07 April 2022
By incorporating the domain knowledge into a machine learning model, KGML-ag overcomes the well-known limitations of process-based models due to insufficient representations and constraints, and unlocks the “black box” of machine learning models. Therefore, KGML-ag can outperform existing approaches on capturing the hot moment and complex dynamics of N2O flux. This study will be a critical reference for the new generation of modeling paradigm for biogeochemistry and other geoscience processes.
The press release by the University of Minnesota can be found at: https://twin-cities.umn.edu/news-events/new-study-could-help-reduce-agricultural-greenhouse-gas-emissions
Contact: Zhenong Jin (jinzn@umn.edu)
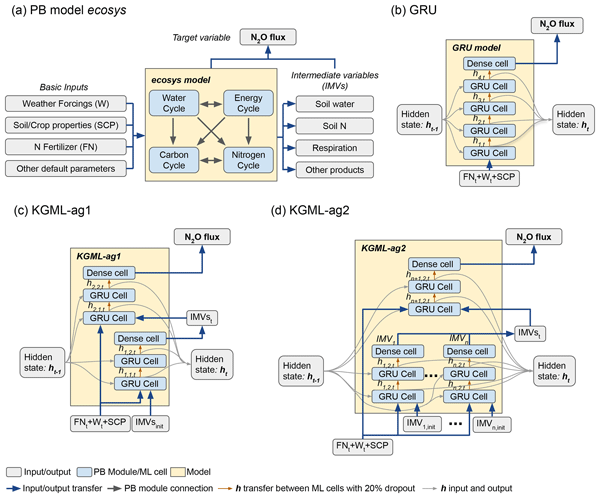